Posted on July 22, 2024 in Photonics Initiative
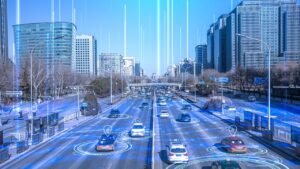
The advance could enhance self-driving cars and reduce energy consumption for technologies requiring intense image processing.
NEW YORK, NY — CUNY ASRC researchers in collaboration with Australian scientists from the ARC Centre of Excellence for Transformative Meta-Optical Systems (TMOS) have developed a new image processing technology that can be used to enhance self-driving cars, minimize drone size and extend their deployment times, and reduce operating costs for these and other technologies. The new advance drastically reduces latency and energy consumption compared to current approaches.
The researchers have created a tunable ultrathin film with a dual-mode of operation that allows a view of either a detailed infrared image or the outline of the image with enhanced edges. By switching between these two modes of operation, it’s possible to more easily detect specific objects in a complex scene such as traffic or forested areas. The advance will enable development of lightweight, ultrafast, and low-energy image processors that can be used for a variety of remote sensing applications, including environmental monitoring and crop surveillance.
The group’s work is detailed in a recently published article in Nature Communications.
Edge detection is an image processing tool that extracts the outline of an object, helping to distinguish objects from their backgrounds. Currently, it’s a digital process that occurs after an image is captured, and requires bulky processors and traditional imaging systems. This form of digital edge detection creates large amounts of data that need to be processed, stored, and transmitted. This is an especially big challenge for self-driving systems, which need to acquire complex scenes with high resolution and process them in real time to recognize humans, street signals, or other objects of relevance.
The analog image filter developed by CUNY ASRC researchers can, on demand, reduce the subject to its outlines prior to capturing the image, drastically reducing the amount of data to be processed for object recognition. It can also switch to an unfiltered, detailed infrared image when required, which is a novel development.
“While a few recent demonstrations have achieved analog edge detection using metasurfaces, the devices demonstrated so far are static in nature,” said the paper’s lead author Michele Cotrufo, assistant professor at the University of Rochester and a former postdoctoral researcher in the lab of Andrea Alù, director of the CUNY ASRC’s Photonics Initiative and distinguished professor at the CUNY Graduate Center. “Their functionality is fixed in time and cannot be dynamically altered or controlled. Yet, the ability to dynamically reconfigure processing operations is key for metasurfaces to be able to compete with digital image processing systems. This is what we have developed.”
The team’s work could result in object recognition systems with less latency and weight, and lower energy consumption. The thin film is only nanometres thick, with a thin layer of the phase change material vanadium dioxide (VO2) embedded within a thicker silicon metasurface. When the temperature of the filter is changed, the VO2 transitions from an insulating state to a metallic one, and the processed image shifts from a filtered outline to an unfiltered infrared image.
“We used a VO2 layer and local heating element as a proof of concept,” said Alù. “Now there’s potential to expand the research to include non-volatile phase change materials, which do not require heating, or to integrate it with an external laser for optically induced heating. The latter scenario may open interesting avenues for all optically reconfigurable nonlinear analogue computation.”
The work was supported by the Air Force Office of Scientific Research MURI program and TMOS.